Groupe de travail MINERS
Data Mining and Machine Learning Team of LIMOS
Responsable communication :
EL CHEIKH Rim
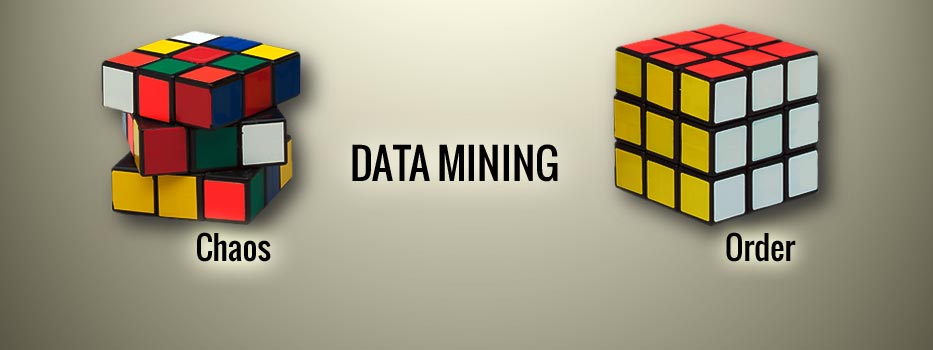
Membres : FALIH Issam - MEPHU NGUIFO Engelbert - MBOUOPDA Michael Franklin - DJIBEROU MAHAMADOU Abdoul Jali - HOSSAIN Sheikh Imran - Jiarui XIE - Benoit ALBERT - Hélène TRAN - ENNAOUI Karima - YEPMO Véronne - HASSAN Md Shahriar - Jocelyn De GOER -
Nouvelle URL de notre site web : https://miners.limos.fr
Talk: Uncertainty-aware and Interpretable Photometric Astronomical Time Series Classification - 18 octobre 2021 - SEMINAIRE
Authors: Michael Franklin MBOUOPDA and Engelbert MEPHU NGUIFO
Abstract: Given the large amount of data generated by today's telescopes such as the LSST one, machine learning has become ineluctably necessary to analyze these data efficiently in order to have a better understanding of the universe. The methods used for collecting these data and the conditions in which the measurement is done are such that the data are imprecise and hence have uncertainty. This uncertainty needs to be taken into account when building machine learning models for this data. Furthermore, interpretable models are required by domain experts in order to be trusted, but also for drawing confident conclusions on the analysis. Unlike time series classification (TSC) which has been highly studied during the last decade, the field of uncertain time series classification (uTSC) is still under-explored. The existing works for uTSC are based on the combination of the 1-Nearest Neighbor (1-NN) classifier and an uncertain similarity measure. However, it has been proved recently that this approach is less effective compared to approaches that perform classification regarding local and/or global features extracted from the time series. In this work, we review the existing uncertain similarity measures and propose two novel ones that are based on f-divergences. For the sake of interpretability, we then combine these uncertain measures with the shapelet classification approach in order to classify the PLAsTiCC dataset.
Date: 19 October 2021, at Lyon, France
http://www.madics.fr/actions/bigdata4astro/
Kilichi Party - 21 septembre 2021 - GENERAL
We enjoyed eating Kilichi today. Kilichi is a dried meat on which different spices have been added. It is mainly found in Niger, Nigeria and Cameroon. Some of us were discovering that meat, for others, it was a great souvenir of what they used to eat.
Check out the Wikipedia Kilichi's page
Measuring consistency for fuzzy logic theories by Prof. Manuel Ojeda-Aciego form University of Malaga - 11 septembre 2021 - SEMINAIRE
Bio Manuel Ojeda-Aciego
17 September 2021, 10 am
Early diagnosis of Lyme disease by recognizing Erythema Migrans skin lesion from images utilizing deep learning techniques: DSAA 2021 PhD Track - 18 août 2021 - PUBLICATION
Authors: Sk Imran Hossain, Engelbert Mephu Nguifo and Jocelyn de Goër de Herve
Abstract: Lyme disease is one of the most common infectious vector-borne diseases in the world. We extensively studied the effectiveness of convolutional neural networks for identifying Lyme disease from images. Our research plan includes multimodal learning, automation of skin hair mask generation and improving neural architecture search.
Uncertain Time Series Classification - IJCAI 2021 - 16 août 2021 - PUBLICATION
Time series analysis has gained a lot of interest during the last decade with diverse applications in a large range of domains such as medicine, physic, and industry. The field of time series classification has been particularly active recently with the development of more and more efficient methods. However, the existing methods assume that the input time series is free of uncertainty. However, there are applications in which uncertainty is so important that it can not be neglected. This project aims to build efficient, robust, and interpretable classification methods for uncertain time series.
Les académiciens, qu’en pensent-ils ? - 11 août 2021 - GENERAL
Dernière étape de notre parcours, l’Observatoire de la Maturité Data touche à sa fin. Après vous avoir acculturé, donné le retour d’expérience d’un répondant, expliqué ce qu’est la maturité data pour des professionnels, on s’attarde aujourd’hui sur le point de vue de Monsieur Engelbert Mephu Nguifo. Maître de conférence et professeur à l’Université Clermont-Auvergne.
Accepted for Oral presentation at CAP'2021: Scalable and Accurate Subsequence Transform - 19 mai 2021 - PUBLICATION
Authors: Michael Franklin MBOUOPDA and Engelbert MEPHU NGUIFO
Abstract : Time series classification using phase-independent subsequences called shapelets is one of the best approaches in the state of the art. This approach is especially characterized by its interpretable property and its fast prediction time. However, given a dataset of n time series of length at most m, learning shapelets requires a computation time of O(n 2 m 4) which is too high for practical datasets. In this paper, we exploit the fact that shapelets are shared by the members of the same class to propose the SAST (Scalable and Accurate Subsequence Transform) algorithm which is interpretable, accurate and more faster than the actual state of the art shapelet algorithm. The experiments we conducted on the UCR archive datasets shown that SAST is more accurate than the state of the art Shapelet Transform algorithm on many datasets, while being significantly more scalable.
Model overview
Paper's link: https://hal.uca.fr/hal-03087686
The 2021 ICML Workshop on Computational Biology - 19 mai 2021 - SEMINAIRE
Important Dates
Deadline for submissions : May 25th 2021 (extended from May 22nd 2021)
Reviewer deadline : June 11th 2021
Notification of acceptance : June 14th, 2021
Video recording deadline : June 26th, 2021
Camera-ready deadline : July 16th, 2021
Workshop date : July 24th 2021
The ICML Workshop on Computational Biology (WCB) will highlight how machine learning approaches can be tailored to making both translational and basic scientific discoveries with biological data. Practitioners at the intersection of computation, machine learning, and biology are in a unique position to frame problems in biomedicine, from drug discovery to vaccination risk scores, and the Workshop will showcase such recent research. Commodity lab techniques lead to the proliferation of large complex datasets, and require new methods to interpret these collections of high-dimensional biological data, such as genetic sequences, cellular features or protein structures, and imaging datasets. These data can be used to make new predictions towards clinical response, to uncover new biology, or to aid in drug discovery.
This workshop aims to bring together interdisciplinary machine learning researchers working in areas such as computational genomics; neuroscience; metabolomics; proteomics; bioinformatics; cheminformatics; pathology; radiology; evolutionary biology; population genomics; phenomics; ecology, cancer biology; causality; representation learning and disentanglement to present recent advances and open questions to the machine learning community. We especially encourage interdisciplinary submissions that might not neatly fit into one of these categories.
More info: https://icml-compbio.github.io/#OC
BOOK: Artificial Intelligence What is it, exactly ? - 28 avril 2021 - PUBLICATION
Compressed k-Nearest Neighbors Classification for Evolving Data Streams - 15 avril 2021 - SEMINAIRE
Journée Perspectives et Défis de l'IA (PDIA) - 2 avril 2021 - SEMINAIRE
L’Association Française pour l’Intelligence Artificielle (AFIA) organise sa septième journée PERSPECTIVES ET DEFIS DE l’IA sur le thème de l’EXPLICABILITE.
L’utilisation des systèmes d’apprentissage et d’aide à la décision est devenue courante. L’étude de la fiabilité et de la précision des systèmes concernés est devenue un sujet d’intérêt majeur, et le besoin de comprendre comment de tels systèmes fonctionnent, apprennent ou prennent des décisions est devenu primordial. L’objectif de cette journée est d’étudier et de discuter toutes ces questions, et de rassembler les chercheurs qui s’y intéressent.
La journée est construite autour d’exposés accessibles, de retours d’expériences et de tables rondes favorisant une grande interaction.
Plus d'info ici: https://afia.asso.fr/pdia21/
Photographs from IJCAI 2020 - Yokohama (virtual) - 18 janvier 2021 - GENERAL
Three papers accepted at the national conference EGC'2020 - 30 novembre 2020 - PUBLICATION
GPoID : Extraction de Motifs Graduels pour les Bases de Données Imprécises
By: Michael Chirmeni Boujike, Jerry Lonlac, Norbert Tsopze and Engelbert Mephu Nguifo
Apport de l'entropie pour les c-moyennes floues sur des données catégoriques (French version of Fuzz-IEEE'2020)
By: Abdoul Jalil Djiberou Mahamadou, Violaine Antoine, Engelbert Mephu Nguifo and Sylvain Moreno
Ontology-based data integration in a distributed context of coalition air missions
By: Karima Ennaoui, Mathieu Faivre, Md Shahriar Hassan, Christophe Rey, Lauren Dargent, Hervé Girod and Engelbert Mephu Nguifo
Accepted Paper at ICDMW 2020: Uncertain Time Series Classification with Shapelet Transform - 16 novembre 2020 - PUBLICATION
Authors: Michael F. MBOUOPDA and Engelbert MEPHU NGUIFO
Abstract: Time series classification is a task that aims at classifying chronological data. It is used in a diverse range of domains such as meteorology, medicine and physics. In the last decade, many algorithms have been built to perform this task with very appreciable accuracy. However, applications where time series have uncertainty has been under-explored. Using uncertainty propagation techniques, we propose a new uncertain dissimilarity measure based on Euclidean distance. We then propose the uncertain shapelet transform algorithm for the classification of uncertain time series. The large experiments we conducted on state of the art datasets show the effectiveness of our contribution. The source code of our contribution and the datasets we used are all available on a public repository.
Model overview
A novel algorithm for searching frequent gradual patterns from an ordered data set - 8 octobre 2020 - PUBLICATION
Accepted Paper at WUML2020 (workshop at ECMLPKDD 2020): Classification of Uncertain Time Series by Propagating Uncertainty in Shapelet Transform - 24 juillet 2020 - PUBLICATION
Author: Michael F. MBOUOPDA and Engelbert MEPHU NGUIFO
Abstract: Time series classification is a task that aims at classifying chronological data. It is used in a diverse range of domains such as meteorology, medicine and physics. In the last decade, many algorithms have been built to perform this task with very appreciable accuracy. However, the uncertainty in data is not explicitly taken into account by these methods. Using uncertainty propagation techniques, we propose a new uncertain dissimilarity measure based on euclidean distance. We also show how to classify uncertain time series using the proposed dissimilarity measure and shapelet transform, one of the best time series classification methods. An experimental assessment of our contribution is done on the well known UCR dataset.
Accepted Paper at FUZZ-IEEE2020: Categorical fuzzy entropy c-means - 8 mai 2020 - PUBLICATION
Authors: Abdoul Jalil Djiberou Mahamadou, Violaine Antoine and Engelbert Mephu Nguifo and Sylvain Moreno
Abstract: Hard and fuzzy clustering algorithms are part of the partition-based clustering family. They are widely used in real-world applications to cluster numerical and categorical data. While in hard clustering an object is assigned to a cluster with certainty, in fuzzy clustering an object can be assigned to different clusters given a membership degree. For both types of method an entropy can be incorporated into the objective function, mostly to avoid solutions raising too much uncertainties. In this paper, we present an extension of a fuzzy clustering method for categorical data using fuzzy centroids. The new algorithm, referred to as Categorical Fuzzy Entropy (CFE), integrates an entropy term in the objective function. This allows a better fuzzification of the cluster prototypes. Experiments on ten real-world data sets and statistical comparisons show that the new method can efficiently handle categorical data.
Acticle accepté à CNIA2020: Classification des Séries Temporelles Incertaines par Transformation Shapelet - 6 mai 2020 - PUBLICATION
Auteurs: Michael Franklin MBOUOPDA et Engelbert MEPHU NGUIFO
Résumé: La classification des séries temporelles est une tâche qui consiste à classifier les données chronologiques. Elle est utilisée dans divers domaines tels que la météorologie, la médecine et la physique. Plusieurs techniques performantes ont été proposées durant les dix dernières années pour accomplir cette tâche. Cependant, elles ne prennent pas explicitement en compte l’incertitude dans les données. En utilisant la propagation de l’incertitude, nous proposons une nouvelle mesure de dissimilarité incertaine basée sur la distance euclidienne. Nous montrons également comment faire la classification de séries temporelles incertaines en couplant cette mesure avec la méthode de transformation shapelet, l’une des méthodes les plus performantes pour cette tâche. Une évaluation expérimentale de notre contribution est faite sur le dépôt de données temporelles UCR.
Accepted Paper at FUZZ-IEEE 2019: Evidential clustering for categorical data - 6 mai 2020 - PUBLICATION
Author: A. J. Djiberou Mahamadou, V. Antoine, G. J. Christie and S. Moreno
Abstract: Evidential clustering methods assign objects to clusters with a degree of belief, allowing for better representation of cluster overlap and outliers. Based on the theoretical framework of belief functions, they generate credal partitions which extend crisp, fuzzy and possibilistic partitions. Despite their ability to provide rich information about the partition, no evidential clustering algorithm for categorical data has yet been proposed. This paper presents a categorical version of ECM, an evidential variant of k-means. The proposed algorithm, referred to as catECM, considers a new dissimilarity measure and introduces an alternating minimization scheme in order to obtain a credal partition. Experimental results with real and synthetic data sets show the potential and the efficiency of cat-ECM for clustering categorical data.
https://ieeexplore.ieee.org/abstract/document/8858972
NeuroDeRisk - Semi annual meeting - 28 avril 2020 - SEMINAIRE
Semi-annual face-to-face meeting of the European project NeuroDeRisk , initially planned in Brussels, but held in web conference because of COVID-19.
A meeting to discuss the last 6 months deliverables and futur ones.
Nouvel article publié dans la revue Pattern Recognition - 20 mars 2020 - PUBLICATION
Remise des écharpes docteurs 2020 - 4 février 2020 - SEMINAIRE
Nos nouveaux docteurs en informatique Dr. Angeline PLAUD et Dr. Jocelyn DE GOËR, tous deux encadrés par Prof. Engelbert MEPHU NGUIFO
BIOSS : Groupe de travail sur la biologie systémique symbolique - 20 décembre 2018 - PUBLICATION
Groupe de travail financé par le GdrIA, Monsieur Engelbert Nguifo Mephu, a réalisé une présentation Mercredi 19 décembre 2018. Cette présentation avait pour thème :A Novel Computational Approach for Global Alignment for Multiple Biological Networks.
mephu_expose_IA_multiformes_2.pdf - 22 novembre 2018 - PUBLICATION
mephu_expose_IA_multiformes_2.pdf.zip
L’intelligence Artificielle est-elle multiforme ? - 21 novembre 2018 - PUBLICATION
Présentation effectuée à l'Université Ouverte de Clermont-Ferrand
AALTD'18 Accepted Paper - 19 septembre 2018 - PUBLICATION
Time Series Classification with Recurrent Neural Networks
by Denis Smirnov and Engelbert Mephu Nguifo
IMG_2530 - 19 septembre 2018 - PUBLICATION
IMG_2530 - 19 septembre 2018 - PUBLICATION
IMG_2530 - 19 septembre 2018 - PUBLICATION
ECML PhD Forum 2018 Presentation - 26 juillet 2018 - PUBLICATION
Title: Classification of multivariate time series based on bihistograms Authors : Angéline PLAUD, Engelbert Mephu, Jacques Charreyron This paper will be present during the PhD Forum of the ECML conference 10-14 septembre 2018 in Dublin.
Grasp heuristic for time series compression with piecewise aggregate approximation - 22 juillet 2018 - PUBLICATION
Vanel Steve Siyou Fotso, Engelbert Mephu Nguifo, Philippe Vaslin published a new research paper entitled Grasp heuristic for time series compression with piecewise aggregate approximation in Journal : RAIRO, Operations Research
L’intelligence Artificielle est-elle multiforme ? - 3 avril 2018 - PUBLICATION
Paper - FUZZ-IEEE 2018 - 28 mars 2018 - PUBLICATION
Author(s): Jerry Lonlac, Yannick Miras, Aude Beauger, Vincent Mazenod, Jean-Luc Peiry and Engelbert Mephu Nguifo Title: An Approach for Extracting Frequent (Closed) Gradual Patterns Under Temporal Constraint has been accepted for presentation at the FUZZ-IEEE 2018 and for publication in the conference proceedings published by IEEE. This email provides you with all the information you require to complete your paper and submit it for inclusion in the proceedings.
Presentation - Denis Smirnov - Deeplearning - 28 mars 2018 - PUBLICATION
slide - 28 mars 2018 - PUBLICATION
DL_interpretability - 28 mars 2018 - PUBLICATION
Nomination as Senior Member of the ACM - 15 janvier 2018 - PUBLICATION
Dear Engelbert Mephu Nguifo: On behalf of the ACM Senior Member Committee, I am pleased to inform you that your nomination as Senior Member of the ACM has been accepted. The Senior Member Committee gratefully acknowledges your efforts in submitting a nomination for review and hopes that your interest in the advanced member program will continue. As part of your recognition, you will be receiving a certificate and pin, and an annotated membership card with the designation of Senior Member. Your certificate and pin will be mailed to you within three to four weeks. Also, your name will appear on the ACM Senior Member page, http://awards.acm.org/senior-members. On behalf of ACM, we are delighted that you will be among the inductees honored with this designation and wish to congratulate you on this well-deserved recognition. Sincerely, Nancy M. Amato Chair, ACM Senior Member Committee
An Experimental Survey on Big Data Frameworks - 22 novembre 2017 - PUBLICATION
Réunion MINERS du 22/11/2017, W. Inoubli présente une comparaison des outils de Big Data
Slides-meeting-LIMOS-22-11-2017 - 22 novembre 2017 - PUBLICATION
Slides-meeting-LIMOS-22-11-2017.pptx
presentation_miners_22112017 - 22 novembre 2017 - PUBLICATION
Slides-meeting-LIMOS-22-11-2017 - 22 novembre 2017 - PUBLICATION
Slides-meeting-LIMOS-22-11-2017.pdf
Time Series Workshop @ ICML , August 2017 - Sydney, Australia - 20 septembre 2017 - PUBLICATION
Harbin Institute of technology - August 2017 - Shen Zhen, China - 20 septembre 2017 - PUBLICATION
ECMLPKDD, September 2017- SKOPJE, MACEDONIA - 20 septembre 2017 - PUBLICATION
Harbin_institute - 20 septembre 2017 - PUBLICATION
TSW@ICML2017 - 20 septembre 2017 - PUBLICATION
Ecml_pkdd_2017 - 20 septembre 2017 - PUBLICATION
TSW@ICML2017 - 20 septembre 2017 - PUBLICATION
Réunion de l'équipe MINERS 26/27 Juillet 2017 - 27 juillet 2017 - PUBLICATION
[gallery]
WhatsApp Image 2017-07-26 at 18.26.05 - 27 juillet 2017 - PUBLICATION
WhatsApp Image 2017-07-26 at 18.26.04 - 27 juillet 2017 - PUBLICATION
WhatsApp Image 2017-07-26 at 17.12.45 - 27 juillet 2017 - PUBLICATION
WhatsApp Image 2017-07-26 at 17.12.43 - 27 juillet 2017 - PUBLICATION
WhatsApp Image 2017-07-26 at 17.12.41 - 27 juillet 2017 - PUBLICATION
WhatsApp Image 2017-07-26 at 17.12.38 - 27 juillet 2017 - PUBLICATION
WhatsApp Image 2017-07-26 at 17.12.36 - 27 juillet 2017 - PUBLICATION
WhatsApp Image 2017-07-26 at 17.12.34 - 27 juillet 2017 - PUBLICATION
WhatsApp Image 2017-07-26 at 17.12.31 - 27 juillet 2017 - PUBLICATION
WhatsApp Image 2017-07-26 at 17.12.27 - 27 juillet 2017 - PUBLICATION
WhatsApp Image 2017-07-26 at 17.12.25 - 27 juillet 2017 - PUBLICATION
WhatsApp Image 2017-07-26 at 17.12.24 - 27 juillet 2017 - PUBLICATION
WhatsApp Image 2017-07-27 at 13.57.39 - 27 juillet 2017 - PUBLICATION
WhatsApp Image 2017-07-27 at 13.57.39 (1) - 27 juillet 2017 - PUBLICATION
cropped-WhatsApp-Image-2017-07-26-at-18.26.051.jpg - 27 juillet 2017 - PUBLICATION
http://home.isima.fr/miners/wp-content/uploads/2017/07/cropped-WhatsApp-Image-2017-07-26-at-18.26.051.jpg
hoto-Z redshift reconstruction using a constructive multilayer perceptron - 27 avril 2017 - PUBLICATION
On Containment of Triclusters Collections Generated by Quantified Box Operators - 27 avril 2017 - PUBLICATION
Clustering flou non-supervisé sur de grands volumes de séquences d'ADN - 27 avril 2017 - PUBLICATION
TITLE: Clustering flou non-supervisé sur de grands volumes de séquences d'ADN AUTHORS: Alexandre Bazin, Didier Debroas and Engelbert Mephu Nguifo
Mining Triclusters of Similar Values in Triadic Real-Valued Contexts - 15 avril 2017 - PUBLICATION
Dmitry Egurnov, Dmitry Ignatov and Engelbert Mephu Nguifo, "Mining Triclusters of Similar Values in Triadic Real-Valued Contexts" International Conference on Formal Concept Analysis, Rennes, 2017
Vers une stratégie de réduction de la base de clauses apprises fondée sur la relation de dominance - 15 avril 2017 - PUBLICATION
Jerry Lonlac and Engelbert Mephu Nguifo, "Vers une stratégie de réduction de la base de clauses apprises fondée sur la relation de dominance", Treizièmes Journées Francophones de Programmation par Contraintes, Lens, juin 2017.
Big Graph Mining : Frameworks and Techniques - 28 février 2017 - PUBLICATION

aridhi_mephu_slides_part1 - 28 février 2017 - PUBLICATION
aridhi_mephu_slides_part2 - 28 février 2017 - PUBLICATION
Extraction de Motifs Graduels (Fermés) Fréquents Sous Contrainte de la Temporalité - EGC 2017 - 27 février 2017 - PUBLICATION
Parameter Free Dynamic Time Warping - ROADEF 2017 - 27 février 2017 - PUBLICATION
slides_EGC_Jerry - 27 février 2017 - PUBLICATION
extraction_de_motifs_graduels - 27 février 2017 - PUBLICATION
parameter_free_piecewise_dtw - 27 février 2017 - PUBLICATION
Vanel_presentation_roadef-2017 - 27 février 2017 - PUBLICATION
Vanel_presentation_roadef-2017.pdf
big_graph_mining - 27 février 2017 - PUBLICATION
Dmitrii Egurnov, lauréat of the competition of research papers in Computer Science among Masters students - 8 février 2017 - PUBLICATION
nirs - 8 février 2017 - PUBLICATION
nirs - 8 février 2017 - PUBLICATION
Remise des diplômes de Doctorat, chercheurs de l'équipe MINERS - 30 janvier 2017 - PUBLICATION
[gallery]
20131213_190627 - 30 janvier 2017 - PUBLICATION
20131213_190658 - 30 janvier 2017 - PUBLICATION
20131213_190707 - 30 janvier 2017 - PUBLICATION
20131213_190817-ConvertImage - 30 janvier 2017 - PUBLICATION
Presentation du Pr. Mehpu au seminaire UFMG à BeloHorizonte - 2 décembre 2016 - PUBLICATION
Première partie Deuxième partie
mephu_seminaire_UFMG_BeloHorizonte_021216_compact - 2 décembre 2016 - PUBLICATION
mephu_seminaire_UFMG_BeloHorizonte_021216_compact.pdf
mephu_seminaire_UFMG_BeloHorizonte_021216_part1_compact - 2 décembre 2016 - PUBLICATION
mephu_seminaire_UFMG_BeloHorizonte_021216_part1_compact.pdf
baniere.png - 28 novembre 2016 - PUBLICATION
http://home.isima.fr/miners/wp-content/uploads/2016/11/baniere.png
From Bioinformacs to Astroinformacs curriculum : A French experience - 28 octobre 2016 - PUBLICATION
Engelbert MEPHU NGUIFO BigSkyEarth Cost Action meeting Sorrento (Italy), October 24-‐25, 2016 La présentation est disponible ici : partie 1 partie2
WhatsApp Image 2016-09-01 at 20.05.554 - 28 octobre 2016 - PUBLICATION
WhatsApp Image 2016-09-01 at 20.05.553 - 28 octobre 2016 - PUBLICATION
WhatsApp Image 2016-09-01 at 20.05.552 - 28 octobre 2016 - PUBLICATION
WhatsApp Image 2016-09-01 at 20.05.55 - 28 octobre 2016 - PUBLICATION
mephu_slides_From_Bio_To_Astro_Informatics_part1 - 28 octobre 2016 - PUBLICATION
mephu_slides_From_Bio_To_Astro_Informatics_part1.pdf
mephu_slides_From_Bio_To_Astro_Informatics_part2 - 28 octobre 2016 - PUBLICATION
mephu_slides_From_Bio_To_Astro_Informatics_part2.pdf
Soutenance des étudiants en master année académique 2015/2016 - 8 septembre 2016 - PUBLICATION
[gallery]
Big Graph Mining: Frameworks and Techniques - 26 juillet 2016 - PUBLICATION
Sabeur Aridhi and Engelbert Mephu Nguifo published a new research paper entitled Big Graph Mining: Frameworks and Techniques in Journal: Big Data Research, Elsevier

Papier de Manel présenté à JOBIM - 10 juin 2016 - PUBLICATION
Le papier de Manel a été accepté pour une présentation à JOBIM 2016
workshop : Big Data, Large-Scale Optimization and Applications - 9 juin 2016 - PUBLICATION
This workshop first intends to bring together, on June 06 and 07, mathematicians and computer scientists from Vancouver, British Columbia and Clermont-Ferrand, France, to exchange new ideas and discuss research directions in the fields of big-data analytics and large-scale optimization. Through an industrial day, organized on June 09, the workshop will also gather together mathematicians and computer scientist from both academia and industry to confront challenging problems in industrial big data and large-scale optimization. The goals of this workshop are twofold. First, it aims to set up a collaborative network of Canadian and French researchers in mathematics and computer science. Secondly, it intends to identify promising new research projects with an emphasis on big data, optimization problems of increasingly greater scale and their applications.
MINERS intervient au comité scientifique et pédagogique et au mini-forum d'Exposciences le 26/05/2016 @ Poyldôme @ Clermont-Ferrand - 28 mai 2016 - PUBLICATION
Cette année Alexandre Bazin et Jerry Lonlac deux postdoctorants en informatique appartenant au groupe de travail MINERS ont participé aux journées scientifiques organisées à Clermont-Ferrand.
- Alexandre a animé le comité scientifique et pédagogique le jeudi matin de 9h à 12h, il a échangé avec des jeunes sur leurs projets et la démarche scientifique à utiliser;
- Jerry a animé avec deux autres enseignants-chercheurs de L'UBP le mini-forum le jeudi de 14h30 à 16h00 : il s'agissait d'un échange libre avec les jeunes sur le thème "intelligence artificielle" .
Ce fut un moment privilégié d'échange qui, nous l'espérons, a planté la petite graine de l'amour de la science dans le cœur des jeunes collégiens et lycéens présents./>
wwwAfrica2016 - 21 avril 2016 - PUBLICATION
Aims and scope
wwwAfrica2016 is a special event of www2016 with three main objectives:
- provide a meeting platform for African web developers and researchers;
- stimulate the development of e-Gov, e-Health, e-Education and Smart-cities via the link between African web actors and deciders in African countries;
- stimulate the research publication on web related topics to African issues and help African scientists access to world-class conferences.
Resources are being solicited to support the fees for a limited number of African students and scientists.
Boolean Factors Based Artificial Neural Network - 21 avril 2016 - PUBLICATION
Paper accepted to IJCNN'2016 for Oral presentation International Joint Conference on Neural Networks "Boolean Factors Based Artificial Neural Network" Lauraine Tiogning Kueti, Norbert Tsopze, Cezar Mbiethieu, Engelbert Mephu-Nguifo and Laure Pauline Fotso
Conférence francophone en Apprentissage 2016 - 10 février 2016 - PUBLICATION
Depuis 1999, la conférence francophone sur l’apprentissage automatique (CAp) est le rendez-vous annuel incontournable de la communauté scientifique travaillant dans le domaine de l’Apprentissage Automatique. L'édition 2016 aura lieu à Marseille du 4 au 7 juillet. Les articles doivent être soumis avant le 10 avril 2016 via le système easychair (pas encore ouvert). L’Apprentissage Automatique est une discipline de l’informatique, liée à l'intelligence artificielle, qui s’intéresse au développement de modèles et d’algorithmes permettant à la machine d’évoluer par apprentissage et ainsi de remplir des tâches qu'il est difficile ou impossible de remplir par des moyens algorithmiques plus classiques. L’Apprentissage Automatique est au cœur de la science des données qui a émergé ces dernières années comme un secteur industriel en très forte progression intimement lié à l’explosion des données et des besoins associés (phénomène résumé sous la dénomination de Big Data).
Journées Ouvertes en Biologie, Informatique et Mathématiques - 10 février 2016 - PUBLICATION
La 17ème édition des Journées Ouvertes en Biologie, Informatique et Mathématiques (JOBIM) se déroulera du 28 au 30 juin sur le site Jacques Monod du campus de l'ENS à Lyon. Cette conférence, placée sous l'égide de la Société Française de Bioinformatique (SFBI), constitue le rendez-vous annuel de la communauté francophone en bioinformatique. Le service en ligne de soumission des résumés ouvrira le 1er mars et la clôture des soumissions est fixée au 14 avril. Toutes les soumission doivent être effectuées sur le site de la conférence et nécessitent de disposer au préalable d'un compte sur le service Sciencesconf.org. La procédure de création de compte peut etre accédéee à partir de ce lien.
Award - 26 janvier 2016 - PUBLICATION
Ekaterina was awarded the third prize for her Master's thesis (all disciplines) by the High School of Economics - Russia in 2015. [caption id="attachment_449" align="aligncenter" width="223" caption="Award - Ekaterina"][/caption]
photo_Ekaterina_Award_Master_HSE - 26 janvier 2016 - PUBLICATION
photo_Ekaterina_Award_Master_HSE (1) - 26 janvier 2016 - PUBLICATION
EGC 2016 - 13 janvier 2016 - PUBLICATION
Le 19 janvier 2016, dans le cadre de EGC à Reims, la deuxième journée EXTRACTION ET GESTION DES CONNAISSANCES et INTELLIGENCE ARTIFICIELLE réunit les deux communautés autour du thème des « Données Participatives et Sociales ». Ces données sont au coeur de nouveaux défis tant au niveau de la fouille de données que de l'intelligence artificielle. Les travaux de la littérature sont généralement associés à l'une des deux communautés, sans montrer le lien entre elles. Cet atelier cherche particulièrement à focaliser sur ce lien du point de vue représentation qu'analyse. L’atelier se tient dans le cadre de la conférence EGC 2016 à à IUT de Reims-Chalons-Charleville, Chemin des Rouliers, 51100 Reims. Les inscriptions se font sur le site de la conférence (http://egc2016.univ-reims.fr/index.php/Inscription). Plus d’information sur le programme : http://www.afia.asso.fr/tiki-download_file.php?fileId=268

The miners meeting is scheduled on Thursday, September 24 at 3pm (French time). Room D010 - 22 septembre 2015 - PUBLICATION
For those abroad, here is a link to join the meeting. http://classevirtuelle.univ-bpclermont.fr/connect/3d24328df05891e468444b85263b6957
ICDM 2015 IEEE International Conference on Data Mining - 11 septembre 2015 - PUBLICATION
The IEEE International Conference on Data Mining series (ICDM) has established itself as the world's premier research conference in data mining. It provides an international forum for presentation of original research results, as well as exchange and dissemination of innovative, practical development experiences. The conference covers all aspects of data mining, including algorithms, software and systems, and applications. ICDM draws researchers and application developers from a wide range of data mining related areas such as statistics, machine learning, pattern recognition, databases and data warehousing, data visualization, knowledge-based systems, and high performance computing. By promoting novel, high quality research findings, and innovative solutions to challenging data mining problems, the conference seeks to continuously advance the state-of-the-art in data mining. Besides the technical program, the conference features workshops, tutorials, panels.
Contest Starts: Jun 01, 2015 Paper submission: Jun 03, 2015 Tutorial proposals: Jul 13, 2015 Workshop and demo submission: Jul 20, 2015
SFC 2015 : XXII ÈMES RENCONTRES DE LA SOCIÉTÉ FRANCOPHONE DE CLASSIFICATION 9-11 SEPT. 2015 NANTES (FRANCE) - 6 septembre 2015 - PUBLICATION
PRÉSENTATION La SFC organise chaque année les Rencontres de la Société Francophone de Classification qui ont pour objectifs de présenter des résultats récents, des applications originales en classification ou dans des domaines connexes, de favoriser les échanges scientifiques à l'intérieur de la société et de faire connaître à divers partenaires extérieurs les travaux de ses membres. Soumission L’acceptation des exposés se fait sur des résumés étendus [ici]. La date limite d’envoi est le 13 mai 21 mai 2015. Une publication sous forme de post-actes est prévue. Une publication sous forme de post-actes est prévue dans la Revue des Nouvelles Technologies de l'nformation (RNTI). L'appel à communication pour les post-actes sera fait à l'issue du colloque. Programme
Programme_provisoire - 6 septembre 2015 - PUBLICATION
EDBT 2016 CALL FOR PAPERS - 19 juillet 2015 - PUBLICATION
EDBT 2016 March 15-18, 2016 - Bordeaux, France CALL FOR PAPERS ================ The International Conference on Extending Database Technology is a leading international forum for database researchers, practitioners, developers, and users to discuss cutting-edge ideas, and to exchange techniques, tools, and experiences related to data management. We encourage submissions or research contribution relating to all aspects of data management defined broadly, and particularly encourage work on topics of emerging interest in the research and development communities. Topics of Interest —————————————————— We welcome papers on topics including, but not limited to, the following: o Availability, Reliability, and Scalability o Tuning, Monitoring, Benchmarking and Performance Evaluation o Big Data Storage, Processing and Transformation o Data Curation, Annotation and Provenance o Data Management in Clouds o Complex Event Processing and Data Streams o Data Mining and Knowledge Discovery o Data Warehousing, Large-Scale Analytics, and ETL Tools o Emerging Hardware and In-memory Database Architecture and Systems o Heterogeneous Databases, Data Integration and Interoperability o Middleware and Workflow Management o Parallel, Distributed and Grid Data Management o Privacy, Trust and Security in Databases o Indexing, Query Processing and Optimization o Semantic Web and Knowledge Management o Sensor and Mobile Data Management o Scientific and Statistical Databases o Social Networks and Crowdsourcing o Graph Databases o Spatial, Temporal, and Geographic Databases o Text Databases and Information Retrieval o Semi-Structured and Linked Data Management o Modeling, Mining and Querying User Generated Content o Data Quality o User Interfaces and Data Visualization Research papers will be selected for inclusion in the program on the basis of their originality, significance and rigor. Selected best papers will be considered for submission to a special issue of TODS. Important Dates for Research and Vision Papers —————————————————————————————————————————————— o Abstract submission deadline: September 21, 2015, 4:59pm CET o Paper submission deadline: September 28, 2015, 4:59pm CET o Notification: December 10, 2015 Submission Guidelines ————————————————————— All aspects of the submission and notification process will be handled electronically. All papers should be submitted in electronic format using the conference submission site Research papers should be submitted to the Research Track, while vision papers to the Vision Track. EDBT 2016 submissions are reviewed following a single blind review process, meaning, you do not need to hide authors’ names and affiliations. EDBT Program Committee Chair ————————————————————————————— Evaggelia Pitoura, University of Ioannina, Greece EDBT Program Committee Members ———————————————————————————————— Bernd Amann Universite Pierre et Marie Curie, France Walid Aref Purdue University, USA Sourav S Bhowmick Nayang Technology University, Singapore Michael Bohlen University of Zurich, Switzerland Klemens Bohm Karlsruhe Institute of Technology, Germany Francesco Bonchi Yahoo! Labs, Spain Angela Bonifati Lille 1 University, France Philippe Bonnet ITU, Copenhagen, Denmark Luc Bouganim INRIA, France Nieves Brisaboa Universidad de La Coruna, Spain Reynold Cheng University og Hong Kong Beng Chin Ooi National University of Singapore, Singapore Vassilis Christophides University of Crete, Greece Panos K Chrysanthis University of Pittsburgh, USA Paolo Ciaccia University of Bologna, Italy Philippe Cudre-Mauroux University of Freibourg, Switzerland Bin Cui Peking University, China Khuzaima Daudjee University of Waterloo, Canada Antonios Deligiannakis Technical University of Crete, Greece Elena Ferrari University of Insubria, Italy Peter Fischer Uni Freiburg, Germany Helena Galhardas University of Lisbon. Portugal Johann Gamper Free University of Bolzen-Bolzano, Italy Minos Garofalakis Technical University of Crete, Greece Floris Geerts University of Antwerp, Belgium Jiawei Han UI Urbana Champaign, USA Takahiro Hara Osaka University, Japan Thomas Heinis Imperial College, UK Arantza Illarramendi Universidad del Paes Vasco, Spain George Kollios Boston University, USA Georgia Koloniari University of Macedonia, Greece Yiannis Kotidis Athens University of Business and Economics, Greece Nick Koudas University of Toronto, Canada Georg Lausen Uni Freiburg, Germany Wang-Chien Lee Penn State University, USA Wolfgang Lehner TU Dresden, Germany Hong-Va Leong Hong Kong Polytechnik University, China Roy Levin IBM Research, Israel Feifei Li University of Utah, USA Xuemin Lin University of New South Wales, Australia Eric Lo Honk Kong Polytechnik, China Norman May SAP, Germany Sebastian Michel TU Kaiserslautern, Germany Kjetil Norvag Norwegian University of Science and Technology, Norway Ippokratis Pandis Cloudera, USA Paolo Papotti QCRI, Qatar Marta Patino Polit?cnica de Madrid, Spain Torben B Pedersen University of Aalborg, Denmark Peter Pietzuch Imperial College, UK Maya Ramanath IIT Delhi, India Matthias Renz Ludwig-Maximilians-University, Germany Rodolfo Resende Universidade Federale de Minas Gerais, Brazil Tore Risch Uppsala University, Sweden Pierangela Samarati Universita degli Studi di Milano, Italy Mohamed Sarwat Arizona State University, USA Kai-Uwe Sattler TU Ilmenau, Germany Marc Scholl University of Konstanz, Germany Heiko Schuldt University of Basel, Switzerland Assaf Schuster Technion, Israel Thomas Seidl RWTH Aachen University, Germany Jianwen Su UC Santa Barbara, USA Peter Triantafillou University of Glasgow, UK Yannis Velegrakis University of Trento, Italy Stratis Viglas University of Edinburgh, UK Jef Wijsen University of Mons (UMONS), Belgium Yoshitaka Yamamoto University of Yamanashi, Japan Carlo Zaniolo UCLA, USA Demetrios Zeinalipour-Yazti University of Cyprus, Cyprus Wenjie Zhang University of New South Wales, Australia
Réunion du groupe miner Jeudi16-07-2015 à 14:00 en D010 - 16 juillet 2015 - PUBLICATION
Vous êtes cordialement invités à la réunion de fin d'année du groupe miner qui aura lieu Jeudi le 16-07-2015 à 14H en D010 (ISIMA)
Seminaire LIMOS, Mardi 30 juin à 10h30 en Amphi Garcia - 19 juin 2015 - PUBLICATION
Quand ? -- Mardi 30 juin à 10h30 Où ? -- Amphi Garcia (Batiment E, ISIMA) Quoi ? -- Séminaire donné par le Professeur Philippe LENCA de Telecom Bretagne, Brest Titre : Sur quelques propriétés des mesure de qualité des règles d'association Résumé : Nous présenterons une synthèse, nécessairement partielle, de travaux concernant les mesures de qualité des règles d’association et des règles d’association de classe en présentant les principaux critères d’évaluation des mesures et en illustrant le rôle de chacun de ces critères dans le comportement des mesures. Nous illustrerons le lien qui existe entre les propriétés des mesures sur les critères retenus et leur comportement sur un certain nombre de bases de règles. Une attention particulière sera portée aux propriétés algorithmiques des mesures afin de pouvoir extraire les motifs intéressants en travaillant directement sur la mesure considérée, sans fixer de seuil de support, ce qui permet d’accéder aux pépites de connaissances. Nous exhiberons des conditions algébriques sur la formule d’une mesure qui assurent de pouvoir associer un critère d’élagage à la mesure considérée.
Towards an efficient estimation of ECM parameters - 19 juin 2015 - PUBLICATION
Presentation-Intelligent-ECM.pdf
Réunion du groupe miner Mercredi 17-06-2015 à 14:00 en D010 - 17 juin 2015 - PUBLICATION
-- You are cordially invited to the presentation of Ekaterina and Hayfa , which will take place on Wednesday, June 17, 2015 at 14:00 a.m. in room D010 Topic: Ekaterina :"Towards an efficient estimation of ECM parameters" Hayfa : "A perceptual hash algorithm for indexing and similarity search in a database of DNA sequences"

AAAI-16 — Thirtieth AAAI Conference on Artificial Intelligence - 2 juin 2015 - PUBLICATION
AAAI-16 welcomes submissions reporting research that advances artificial intelligence, broadly conceived. The conference scope includes all subareas of AI, including (but not limited to) traditional topics such as search, machine learning, planning, knowledge representation, reasoning, natural language processing, robotics and perception, and multiagent systems.
Timetable for Authors
- July 1, 2015 – September 10, 2015: Authors register on the AAAI web site
- September 10, 2015: Electronic abstracts due
- September 15, 2015: Electronic papers due
- October 28-30, 2015: Author feedback about initial reviews
- November 12, 2015: Notification of acceptance or rejection
- December 1, 2015: Camera-ready copy due
Etat d'avancement du travail de thèse - 21 mai 2015 - PUBLICATION
Quand ? -- Jeudi 21/05/2015 Où ? -- ISIMA D010 Quoi ? -- Présentation de l'état d'avancement du tavail de thèse de Vanel SIYOU Sujet : Extraction de connaissances et incertitudes à partir de mesures effectuées lors de la locomotion en Fauteuil Roulant Manuel Encadrant : Englebert Mephu-Nguifo et Philippe Vaslin
etatD'avancement-21-05-2015 - 21 mai 2015 - PUBLICATION
etatDavancement-21-05-2015.pdf
IEEE Big Data 2015 Call for Paper - 18 mai 2015 - PUBLICATION
Towards more targeted recommendations in folksonomies - 11 mai 2015 - PUBLICATION
Présentation de Mohamed Nader Jelassi lors de la conférence CaRR@ICIR15 Encadrant : Engelbert Mephu-Nguifo, Sadok Ben Yahia
expose carr 2015 - 11 mai 2015 - PUBLICATION
PFIA 2015 : Rennes, au coeur de l’intelligence de demain - 8 mai 2015 - PUBLICATION
Du 29 juin au 3 juillet, à Rennes, se tiendra l’édition 2015 de Plate-Forme Intelligence Artificielle (PFIA), un événement organisé par Inria et l’AFIA (l’Association Française pour l’Intelligence Artificielle). Communiqué de presse
PFIA 2015 Communiqué de presse - 8 mai 2015 - PUBLICATION
PFIA-2015-Communiqué-de-presse.pdf
ICML - 06/11 July 2015 Lille Grand Palais - 28 avril 2015 - PUBLICATION
ICML is the leading international machine learning conference and is supported by the International Machine Learning Society (IMLS). Important Dates
- 6 Feb. Paper submission deadline
- 27-31 Mar. Author feedback period
- 25 Apr. Decision notification
- 6 jul. Tutorials
- 7-9 jul. Main Conference
- 10-11 Jul. Workshops
IJCAI, Buenos Aires, 27th July 2015 - 10 avril 2015 - PUBLICATION
The aim of this workshop called Bioinformatics and Artificial Intelligence (BAI) is to bring together active scholars and practionners in the frontier of Artificial Intelligence (AI) and Bioinformatics.
Etude de la scabilité des méthodes d'optimisation d'architecture des réseaux de neurones. Application à l'estimation des redshifts photométriques - 9 avril 2015 - PUBLICATION
Etat d'avancement du travail - 09/04/2015 - Nina Bekono Encadrant : Engelbert Mephu-Nguifo Presentation
International Conference on Machine Learning - (11-15) July 2015 - LILLE GRAND PALAIS - 9 avril 2015 - PUBLICATION
ICML is the leading international machine learning conference and is supported by the International Machine Learning Society (IMLS).
Expose - 9 avril 2015 - PUBLICATION
Call for Papers - IEEE DSAA (Data Science and Advanced Annalytics) 2015 - 26 mars 2015 - PUBLICATION
Please find below the Call for Papers for the IEEE 2015 International Conference on Data Science and Advanced Analytics (DSAA'2015), to be held on 19-21 October 2015 in Paris, France. Website
ECMLPKDD 2015 : Call for Papers, Tutorials and Workshops - 20 février 2015 - PUBLICATION
The European Conference on Machine Learning and Principles and Practice of Knowledge Discovery in Databases (ECMLPKDD) will take place in Porto, Portugal, from September 7th to 11th, 2015
Méthodologie de la recherche - 19 février 2015 - PUBLICATION
Réunion MINERS - 13 février 2015 - PUBLICATION
État d'avancement du travail de Thèse de J. DE GOËR
2015-02-12-MINERS.pdf
Réunion MINERS du 12 février 2015 - 13 février 2015 - PUBLICATION
Point d'avancement du travail de Thèse de Jocelyn DE GOËR Titre: "Stockage, indexation et comparaison d’une grande quantité́ de données génomiques à l’aide d’algorithmes de traitement d’images dans un environnement d’exécution NoSQL et GPU" Présenté par: Jocelyn DE GOËR Sous la direction de: Pr. Engelbert Mephu Nguifo et Pr. Myoung-Ah KANG Consulter la présentation au format PDF ICI
mephu_seminaire_UQAM_LATECE_10dec14_vf.compressed - 11 décembre 2014 - PUBLICATION
mephu_seminaire_UQAM_LATECE_10dec14_vf.compressed.pdf
Fouille de motifs et Préférences - 10 décembre 2014 - PUBLICATION
Contexte : dixième séminaire de l'automne 2014 du LATECE (Laboratory for research on Technology on Ecommerce; Montreal, Quebec, Canada) Orateur : M. Engelbert Mephu Nguifo, professeur d'informatique à l'université Blaise Pascal (Clermont-Ferrand II, France) Titre : Fouille de motifs et Préférences Résumé Pattern mining is still a challenging task in data mining and machine learning, with many applications in biology, physics, chemistry, marketing, etc. One of the bottleneck in such problem comes from the huge number of output generated by any of the several standard algorithms. Introducing user preferences is a direction to tackle such limitations. In this talk, I will review state of the art on preferences in pattern mining. I will put more focus on skyline pattern mining, and more precisely on undominated association rules slides de la présentation qui a eu lieu Mer. 10 Décembre 2014 à 12:15 au PK 5115
Mephu_Debroas_CPER2014-appelCandidature-PostDoc - 22 octobre 2014 - PUBLICATION
Mephu_Debroas_CPER2014-appelCandidature-PostDoc.pdf
ALLOCATION POST-DOCTORALE – 18 mois - 22 octobre 2014 - PUBLICATION
Étude de la biosphère rare microbienne par une approche in silico : nouvelle méthode de classification ensembliste et modélisation La détermination de la structure des communautés (richesse, abondance, diversité, composition) d'un écosystème est un enjeu central en écologie et donc en écologie microbienne. Elle repose sur la détermination des OTUs (i.e. espèces microbienne). Or, cette détermination varie en fonction des méthodes de classification et n'est pas associée à une probabilité d’appartenance à une classe. La difficulté réside généralement soit dans le langage de représentation associé, soit dans le mécanisme d’inférence mis en œuvre. Le LMGE peut être considéré par la nature de sa production scientifique comme un laboratoire leader au niveau international dans la description de la biosphère rare. Le LIMOS a développé ces dernières années des méthodes originales pour traiter le problème de la classification non supervisée en présence d’incertitudes, et pour la prise en compte des préférences de l’utilisateur pour l’extraction de connaissances. L'association de l'expertise d'un laboratoire reconnu dans l'utilisation du séquençage haut débit pour étudier les communautés microbiennes (LMGE) à celle d'un laboratoire d'informatique (LIMOS) est un atout original dans ce domaine de recherche. La diffusion du travail se fera sous la forme de communications académiques. Les avancées technologiques (i.e. classification) seront intégrées au site web ePANAM en cours d'élaboration. La mise en place de tels sites internet a un fort impact sur la communauté scientifique et donc sur la promotion du savoirfaire d'une région comme le montre l'utilisation du site METAVIR dans le monde entier. La coordination sera assurée par Engelbert Mephu-Nguifo et Didier Debroas et se traduira par des réunions régulières organisées par le post-doctorant recruté. Une Présentation du sujet de recherche, des objectifs à atteindre et du calendrier prévisionnel de réalisation est disponible ici
THE 19TH PACIFIC-ASIA CONFERENCE ON KNOWLEDGE DISCOVERY AND DATA MINING - 22 septembre 2014 - PUBLICATION
The Pacific-Asia Conference on Knowledge Discovery and Data Mining (PAKDD) is a leading international conference in the areas of knowledge discovery and data mining (KDD).
2015 SIAM International Conference on DATA MINING - 22 septembre 2014 - PUBLICATION
The SDM conference provides a venue for researchers who are addressing these problems to present their work in a peer-reviewed forum. It also provides an ideal setting for graduate students and others new to the field to learn about cutting-edge research by hearing outstanding invited speakers and attending presentations and tutorials (included with conference registration). A set of focused workshops is also held on the last day of the conference. The proceedings of the conference are published in archival form, and are also made available on the SIAM web site.
Conférences 2014 - 26 août 2014 - PUBLICATION
Neural Information Processing Systems Foundation 2014 The European Conference on Machine Learning and Principles and Practice of Knowledge Discovery in Databases 2014 ECCB'14, the 13th European Conference on Computational Biology
graphx - 19 juillet 2014 - PUBLICATION
Etat d'avancement du travail - 19 juillet 2014 - PUBLICATION
Présenté par: Takwa Ben Smida Sous la direction de: Dr. Sabeur Aridhi Sous la supervision de : Pr. Engelbert Mephu Nguifo présentation
CLA Presentation_GRISSA - 15 juillet 2014 - PUBLICATION
Boolean factors as a means of clustering of interestingness measures of association rules - 15 juillet 2014 - PUBLICATION
Authors: Radim Belohlavek, Dhouha Grissa, Sylvie Guillaume, Engelbert Mephu Nguifo, Jan Outrata presentation
Présentation_PetaSky Cyrine2_partie1 - 12 juillet 2014 - PUBLICATION
Présentation_PetaSky-Cyrine2_partie1.pdf
Présentation_PetaSky Cyrine2_partie2 - 12 juillet 2014 - PUBLICATION
Présentation_PetaSky-Cyrine2_partie2.pdf
Présentation_PetaSky Cyrine2_partie3 - 12 juillet 2014 - PUBLICATION
Présentation_PetaSky-Cyrine2_partie3.pdf
ESTIMATION DES REDSHIFTS PHOTOMERIQUES AVEC LES RESEAUX DE NEURONES - 12 juillet 2014 - PUBLICATION
Présentée par : Cyrine AROURI Participants : Engelbert MEPHU NGUIFO, Cécile ROUCELLE, Gaëlle BONNET LOOSLI, Sabeur ARIDHI présentation : partie 1 présentation : partie 2 présentation : partie 3
Presentation_miner - 11 juillet 2014 - PUBLICATION
Modèle paramétrique et non paramétrique de locomotion en Fauteuil Roulant Manuel - 11 juillet 2014 - PUBLICATION
communityDection - 8 juillet 2014 - PUBLICATION
analyseDiversiteMicrobienneParSequencage_1 - 8 juillet 2014 - PUBLICATION
analyseDiversiteMicrobienneParSequencage_1.pdf
analyseDiversiteMicrobienneParSequencage_2 - 8 juillet 2014 - PUBLICATION
analyseDiversiteMicrobienneParSequencage_2.pdf
analyseDiversiteMicrobienneParSequencage_3 - 8 juillet 2014 - PUBLICATION
analyseDiversiteMicrobienneParSequencage_3.pdf
Aridhi et al., 2014_1 - 8 juillet 2014 - PUBLICATION
Aridhi et al., 2014_2 - 8 juillet 2014 - PUBLICATION
A novel MapReduce-based approach for distributed frequent subgraph mining - 8 juillet 2014 - PUBLICATION
Miners Seminar on July 8, 2014 - 8 juillet 2014 - PUBLICATION
The following master's students will give a 20-30mn talk (French or English). 09:30am- Takwa Ben Smida 10:00am- Vanel Steve Siyou Fotso
11:00am- Andrey Shestakov 11:30am- Cyrine Arouri 12:00am- Nestor Koueya (distance talk) 12:30am- Lunch 02:00 - 05:00pm- Opening hours for PhD talk proposal Najwa TAIB, Jocelyn DE GOER, Dhouha GRISSA
Analyse de la diversité microbienne par séquençage massif - 8 juillet 2014 - PUBLICATION
Auteur : Najwa TAIB presentation, partie 1 presentation, partie 2 presentation, partie 3
Semi-average criterion in community detection problems - 8 juillet 2014 - PUBLICATION
Author : Shestakov Andrey Scientific Supervisors: Boris Mirkin (HSE), Engelbert Mephu Nguifo (LIMOS) file : community detection
exposeIC5-5-14 - 16 juin 2014 - PUBLICATION
exposeIC5-5-14 - 16 juin 2014 - PUBLICATION
exposeIC5-5-14 - 16 juin 2014 - PUBLICATION
exposeIC5-5-14 - 16 juin 2014 - PUBLICATION
exposeIC5-5-14 - 16 juin 2014 - PUBLICATION
Vers des recommandations plus personnalisées dans les folksonomies - 16 juin 2014 - PUBLICATION
Plusieurs approches ont été proposées dans la littérature pour personnaliser les recommandations dans les folksonomies. Dans ce papier, nous considérons une nouvelle dimension dans les folksonomies comme information supplémentaire pour offrir aux utilisateurs une recommandation plus ciblée et mieux conforme à leurs besoins. Cela passe par un regroupement des utilisateurs ayant des intérêts communs sous forme de structures appelées quadri-concepts. Notre approche, dans laquelle nous répondons également au challenge de cold start, est ensuite évaluée sur deux jeux de données du monde réel, MovieLens et BookCrossing. Cette évaluation comprend une mesure de la précision et du rappel, une évaluation sociale ainsi que plusieurs métriques d'évaluation comme la diversité, la couverture ou la scalabilité. exposeIC5-5-14.
Talk at French conference on DataWarehouse 2014 - 2 juin 2014 - PUBLICATION
Nestor Koueya, Sandro Bimonte, Engelbert Mephu Nguifo: Une nouvelle approche d’estimation pour les entrepôts de données multi-granulaires incomplètes. EDA 2014, Vichy (France)
mephu_panelSession_NICST2014 - 28 mai 2014 - PUBLICATION
mephu_panelSession_NICST2014_v3.pdf
Presentation of Engelbert Mephu Nguifo - NICST 2014 workshop, Weihai (China) - Panel session - 28 mai 2014 - PUBLICATION
Title : Smarter Compuing & ICT for Sustainable Development of Human Society and Industry in Big Data Environment mephu_panelSession_NICST2014
Talk of Sabeur Aridhi - Big Data Forum - 19 mai 2014 - PUBLICATION
Title : Mining large datasets : case of mining frequent subgraph in the cloud Date : May 16, 2014
Paper - To appear in "Information Systems" - 19 mai 2014 - PUBLICATION
S. Aridhi, L. d'Orazio, M. Maddouri and E. Mephu Nguifo. Density-based data partitioning strategy to approximate large-scale subgraph mining. Information Systems, Elsevier, 2013, ISSN 0306-4379, http://dx.doi.org/10.1016/j.is.2013.08.005.
Paper - Accepted in "Technique et science informatiques" - 19 mai 2014 - PUBLICATION
S. Aridhi, L. d'Orazio, M. Maddouri and E. Mephu Nguifo. Un partitionnement basé sur la densité de graphe pour approcher la fouille distribuée de sous-graphes fréquents. Technique et Science Informatiques, Lavoisier.
Paper - Accepted in "RFIA 2014" for oral presentation - 19 mai 2014 - PUBLICATION
S. Aridhi, L. d'Orazio, M. Maddouri et E. Mephu Nguifo. A Novel MapReduce-based approach for distributed frequent subgraph mining. 19ème congrès national sur la Reconnaissance de Formes et l'Intelligence Artificielle (RFIA'14), Rouen, France, 2014.
expose_26-03-14 - 10 avril 2014 - PUBLICATION
Towards more targeted recommendations in folksonomies - 7 avril 2014 - PUBLICATION
Towards more targeted recommendations in folksonomies - 7 avril 2014 - PUBLICATION
expose_26-03-14 Recommender systems are now popular both commercially as well as within the research community, where many approaches have been suggested for providing recommendations. Folksonomies’ users are sharing items (e.g.,movies,books,bookmarks, etc.) by annotating them with freely chosen tags. Within the Web 2:0 age, users become the core of the system since they are both the contributors and the creators of the information. In this respect, it is of paramount importance to intercept their needs by considering their respective profiles to use this information for providing a more targeted recommendation. In this paper, we consider users’ profile as a new dimension of a folksonomy classically composed of three dimensions <users,tags,ressources> and propose an approach to group users with close profiles and interests through quadri-concepts. Then, we use such structures in order to propose our personalized recommendation system of users, tags and resources. Carried out extensive experiments on two real-life datasets,i.e.,MOVIELENS and BOOKCROSSING highlight encouraging results in terms of precision as well as a good social evaluation. Moreover, we study some of the key assessment metrics such as coverage, diversity and scalability.
ClaSeek_Luckas - 21 février 2014 - PUBLICATION
Talk of PhD student Lukas Havrlant - 18 février 2014 - PUBLICATION
Title: Search engine based on FCA
bitvector_Lukas - 15 février 2014 - PUBLICATION
Slides - 15 février 2014 - PUBLICATION
Presentation of Lukas Havrlant (Palacky University of Olomouc) - 15 février 2014 - PUBLICATION
Title: Bit-vector encoding and matrix decomposition Date: Febrary 12, 2014 Slides
Presentation of Sabeur Aridhi - 15 février 2014 - PUBLICATION
Title: Distribued graph mining and cloud computing Date: Ferbrary 04, 2014
ECAI'14, The Twenty-first European Conference on Artificial Intelligence, Prague, Czech Republic, 18-22 aout 2014 - 27 décembre 2013 - PUBLICATION
The Twenty-first European Conference on Artificial Intelligence 18-22 August 2014, Prague, Czech Republic http://www.ecai2014.org The biennial European Conference on Artificial Intelligence (ECAI) is Europe's premier archival venue for presenting scientific results in AI. Organised by the European Coordinating Committee for AI (ECCAI), the ECAI conference provides an opportunity for researchers to present and hear about the very best research in contemporary AI. As well as a full programme of technical papers, ECAI'14 will include the Prestigious Applications of Intelligent Systems conference (PAIS), the Starting AI Researcher Symposium (STAIRS), the International Web Rule Symposium (RuleML) and an extensive programme of workshops, tutorials, and invited speakers. (Separate calls are issued for PAIS, STAIRS, tutorials, and workshops.)ECAI'14 will be held in the beautiful and historic city of Prague, the capital of the Czech Republic. With excellent opportunities for sightseeing and gastronomy, Prague promises to be a wonderful venue for a memorable conference. This call invites the submission of papers and posters for the technical programme of ECAI'14. High-quality original submissions are welcome from all areas of AI; the following list of topics is indicative only. - Agent-based and Multi-agent Systems - Constraints, Satisfiability, and Search - Knowledge Representation, Reasoning, and Logic - Machine Learning and Data Mining - Natural Language Processing - Planning and Scheduling - Robotics, Sensing, and Vision - Uncertainty in AI - Web and Knowledge-based Information Systems - Multidisciplinary Topics Both long (6-page) and short (2-page) papers can be submitted. Whereas long papers should report on substantial research results, short papers are intended for highly promising but possibly more preliminary work. Short papers will be presented in poster form. Rejected long papers will be considered for the short paper track. Submitted papers must be formatted according to ECAI'14 guidelines and submitted electronically through the ECAI'14 paper submission site. Full instructions including formatting guidelines and electronic templates are available on the ECAI'14 website. Paper submission: 1 March 2014 Author feedback: 14-18 April 2014 Notification of acceptance/rejection: 9 May 2014 Camera-ready copy due: 30 May 2014 The proceedings of ECAI'14 will be published by IOS Press. Best papers go AIJ The authors of the best papers (and runner ups) of ECAI'14 will be invited to submit an extended version of their paper to the Artificial Intelligence Journal.
PhD Thesis Defense of Wajdi Dhifli - 10 décembre 2013 - PUBLICATION
Place: ISIMA, Campus des Cézeaux (Clermont-Ferrand), Room A102 Date: Wednesday December 11th 2013 at 2:30pm. Examiners Board : -Reviewers :
- Pr. Mohammed Javeed Zaki (Rensselaer Polytechnic Institute, USA)
- Pr. Abdoulaye Baniré Diallo (Université du Québec à Montréal, Canada)
- Pr. Jan Ramon (Katholieke Universiteit Leuven, Belgium)
- DR. David W. Ritchie (INRIA, Nancy, France)
- DR. Jean Sallantin (LIRMM, Montpellier, France)
- DR. Jean-François Gibrat (INRA, Jouy-en-Josas, France)
- Dr. Annegret Wagler (LIMOS, Clermont-Ferrand, France)
PhD Thesis Defense of Sabeur Aridhi - 2 décembre 2013 - PUBLICATION
Title:Distributed Subgraph Mining in the Cloud Place:ISIMA, Campus des Cézeaux (Clermont-Ferrand), Room E005 Date: Friday November 29th at 9am. Jury members: Reviewers: Pr. Anne LAURENT, LIRMM, University of Montpellier 2, France Pr. Takeaki UNO, National Institute of Informatics, Japan Examiners: Pr. Jérome DARMONT, ERIC, University of Lyon 2, France Pr. Mohamed Mohsen GAMMOUDI, University of Manouba, Tunisia Co-Supervisors: Dr. Laurent D'ORAZIO, LIMOS, University of Clermont Ferrand II, France. Pr. Mondher MADDOURI, LIPAH, Université of Manouba, Tunisia Supervisor: Pr. Engelbert MEPHU NGUIFO, LIMOS, University of Clermont Ferrand II, France. Abstract: Recently, graph mining approaches have become very popular, especially in certain domains such as bioinformatics, chemoinformatics and social networks. One of the most challenging tasks in this setting is frequent subgraph discovery. This task has been highly motivated by the tremendously increasing size of existing graph databases. Due to this fact, there is urgent need of efficient and scaling approaches for frequent subgraph discovery especially with the high availability of cloud computing environments. This thesis deals with distributed frequent subgraph mining in the cloud. First, we provide the required material to understand the basic notions of our two research fields, namely graph mining and cloud computing. Then, we present the contributions of this thesis. In the first axis, we propose a novel approach for large-scale subgraph mining, using the MapReduce framework. The proposed approach provides a data partitioning technique that consider data characteristics. It uses the densities of graphs in order to partition the input data. Such a partitioning technique allows a balanced computational loads over the distributed collection of machines and replace the default arbitrary partitioning technique of MapReduce. We experimentally show that our approach decreases significantly the execution time and scales the subgraph discovery process to large graph databases. In the second axis, we address the multi-criteria optimization problem of tuning thresholds related to distributed frequent subgraph mining in cloud computing environments while optimizing the global monetary cost of storing and querying data in the cloud. We define cost models for managing and mining data with a large scale subgraph mining framework over a cloud architecture. We present an experimental validation of the proposed cost models in the case of distributed subgraph mining in the cloud.
Top Research Conferences in Data Mining, Data Science - 24 novembre 2013 - PUBLICATION
Here are the top 10 research conferences, based on the last 10 years data. The leading conference is - KDD (www.kdd.org), both by Field rating and by Citations/Publication. http://www.kdnuggets.com/2013/11/top-conferences-data-mining-data-science.html?goback=%2Eanb_160888_*2_*1_*1_*1_*1_*1#%21 Here is a full list of 38 conferences in data mining. Another ranking of conferences is Google Scholar: Top publications - Data Mining & Analysis
Exposé de Jacqueline: le lundi 14/10/2013 - 11 octobre 2013 - PUBLICATION
Program : Summer School MLSS'13 - 13 septembre 2013 - PUBLICATION
Program : Summer School MLSS'13
program-4.pdf
MLSS'13: Machine Learning Summer School 2013 - 13 septembre 2013 - PUBLICATION
Machine Learning Summer School 2013 @ Al Hambra Hotel, Hammamet, Tunisia September 16-18, 2013 Program : Summer School MLSS'13 Prof. Engelbert Mephu Nguifo will give a talk @ MLSS'13 on Tuesday the 17th of September. The talk is about " Machine learning: Paradigms and Lessons" and will be held in the plenary session between 8:00 and 9:30 am.
séminaire Dr. Haïtham Sghaier (HDR) 12 septembre - 15h30 - Amphi Bruno Garcia - 12 septembre 2013 - PUBLICATION
Jeudi 12 septembre - 15h30 - Amphi Bruno Garcia Title: Top-down computational biology and biochemical methods to predict the evolution of ionizing-radiation-resistant prokaryotes Dr. Haïtham Sghaier, HDR Research Unit UR04CNSTN01 "Medical and Agricultural Applications of Nuclear Techniques", National Center for Nuclear Sciences and Technology (CNSTN), Sidi Thabet Technopark, 2020 Sidi Thabet, Tunisia sghaier.haitham@gmail.com, haitham.sghaier@cnstn.rnrt.tn Abstract Since the beginning of their discovery some 55 years ago, the origin of ionizing-radiation-resistant prokaryotes (IRRP) has been under debate. IRRP were regarded as representing a scattered group from a phylogenetic perspective, urging the notion that these microorganisms emerged through convergent evolution. Despite this provocative hypothesis, the formulation of other theories, and pertinent discoveries, the evolution of IRRP remains either incompletely understood or profoundly misinterpreted. In this presentation, I highlight and elaborate on these issues and discuss top-down computational biology and biochemical methods to decipher the evolution of IRRP and the nature of their ancestor(s).
Smoothing 3D protein structure motifs through graph mining and amino-acids similarities - 14 juillet 2013 - PUBLICATION
@JOBIM 2013
Jobim13.pdf
@JOBIM 2013 : Smoothing 3D protein structure motifs through graph mining and amino-acids similarities - 14 juillet 2013 - PUBLICATION
Wajdi Dhifli, Rabie Saidi, Engelbert Mephu Nguifo. Smoothing 3D protein structure motifs through graph mining and amino-acids similarities. Journées Ouvertes en Biologie, Informatique et Mathématiques (JOBIM), Toulouse, France 2013. Abstract: One of the most powerful techniques to study proteins is to look for recurrent fragments (also called substructures), then use them as patterns to characterize the proteins under study. Although protein sequences have been extensively studied in the literature, studying protein three-dimensional (3D) structures can reveal relevant structural and functional information which may not be derived from protein sequences alone. An emergent trend consists in parsing proteins 3D structures into graphs of amino acids. Hence, the search of recurrent substructures is formulated as a process of frequent sub-graph discovery where each subgraph represents a 3D-motif. In this scope, several efficient approaches for frequent 3D-motifsdiscovery have been proposed in the literature. However, the set of discovered 3D-motifs is too large to be efficiently analyzed and explored in any further process. In this paper, we propose a novel pattern selection approach that shrinks the large number of discovered frequent 3D-motifs by selecting the representative ones. Existing pattern selection approaches do not exploit the domain knowledge. Yet, in our approach we incorporate the evolutionary information of amino acids defined in the substitution matrices in order to select the representative 3D-motifs. We show the effectiveness of our approach on a number of real datasets. The results issued from our experiments show that our approach detects relations between patterns that current subgraph selection approaches fail to detect, and that it is able to considerably decrease the number of motifs while enhancing their interestingness.
Call for papers: NIPS 2013 - 22 avril 2013 - PUBLICATION
Neural Information Processing Systems Conference and Workshops December 5-10, 2013 Lake Tahoe, Nevada, USA http://nips.cc/Conferences/2013/ Deadline for Paper Submissions: Friday, May 31, 2013, 11 pm Universal Time (4 pm Pacific Daylight Time). Submit at: https://cmt.research.microsoft.com/NIPS2013/ Submissions are solicited for the Twenty-Seventh Annual Conference on Neural Information Processing Systems, an interdisciplinary conference that brings together researchers in all aspects of neural and statistical information processing and computation, and their applications. The conference is a highly selective, single track meeting that includes oral and poster presentations of refereed papers as well as invited talks. The 2013 conference will be held on December 5-8 at Lake Tahoe, Nevada. One day of tutorials (December 5) will precede the main conference, and two days of workshops (December 9-10) will follow it at the same location. Note that differently from previous years, the conference will start on a Thursday. Submission process: Electronic submissions will be accepted until Friday, May 31, 2013, 11 pm Universal Time (4 pm Pacific Daylight Time). As was the case last year, final papers will be due in advance of the conference. However, minor changes such as typos and additional references will still be allowed for a certain period after the conference. Reviewing: As in previous years, reviewing will be double-blind: the reviewers will not know the identities of the authors. However, differently from previous years, anonymous reviews and meta-reviews of accepted papers will be made public after the end of the review process. Evaluation Criteria: Submissions will be refereed on the basis of technical quality, novelty, potential impact, and clarity. Dual Submissions Policy: Submissions that are identical (or substantially similar) to versions that have been previously published, or accepted for publication, or that have been submitted in parallel to other conferences are not appropriate for NIPS and violate our dual submission policy. Exceptions to this rule are the following: 1. Submission is permitted of a short version of a paper that has been submitted to a journal, but has not yet been published in that journal. Authors must declare such dual-submissions either through the CMT submission form, or via email to the program chairs at program-chairs@nips.cc. It is the authors’ responsibility to make sure that the journal in question allows dual concurrent submissions to conferences. 2. Submission is permitted for papers presented or to be presented at conferences or workshops without proceedings, or with only abstracts published. Previously published papers with substantial overlap written by the authors must be cited so as to preserve author anonymity (e.g. “the authors of [1] prove that …”). Differences relative to these earlier papers must be explained in the text of the submission. It is acceptable to submit to NIPS 2013 work that has been made available as a technical report (or similar, e.g. in arXiv) without citing it. While this could compromise the authors' anonymity, reviewers will be asked to refrain from actively searching for the authors’ identity or disclose to the area chairs if their identity is known to them. The dual-submission rules apply during the NIPS review period which begins May 31 and ends September 5, 2013. Submission Instructions: All submissions will be made electronically, in PDF format. Papers are limited to eight pages, including figures and tables, in the NIPS style. An additional ninth page containing only cited references is allowed. Complete submission and formatting instructions, including style files, are available from the NIPS website, http://nips.cc. Supplementary Material: Authors can submit up to 10 MB of material, containing proofs, audio, images, video, data or source code. Note that the reviewers and the program committee reserve the right to judge the paper solely on the basis of the 9 pages of the paper; looking at any extra material is up to the discretion of the reviewers and is not required. Technical Areas: Papers are solicited in all areas of neural information processing and statistical learning, including, but not limited to: * Algorithms and Architectures: statistical learning algorithms, kernel methods, graphical models, Gaussian processes, Bayesian methods, neural networks, deep learning, dimensionality reduction and manifold learning, model selection, combinatorial optimization, relational and structured learning. * Applications: innovative applications that use machine learning, including systems for time series prediction, bioinformatics, systems biology, text/web analysis, multimedia processing, and robotics. * Brain Imaging: neuroimaging, cognitive neuroscience, EEG (electroencephalogram), ERP (event related potentials), MEG (magnetoencephalogram), fMRI (functional magnetic resonance imaging), brain mapping, brain segmentation, brain computer interfaces. * Cognitive Science and Artificial Intelligence: theoretical, computational, or experimental studies of perception, psychophysics, human or animal learning, memory, reasoning, problem solving, natural language processing, and neuropsychology. * Control and Reinforcement Learning: decision and control, exploration, planning, navigation, Markov decision processes, game playing, multi-agent coordination, computational models of classical and operant conditioning. * Hardware Technologies: analog and digital VLSI, neuromorphic engineering, computational sensors and actuators, microrobotics, bioMEMS, neural prostheses, photonics, molecular and quantum computing. * Learning Theory: generalization, regularization and model selection, Bayesian learning, spaces of functions and kernels, statistical physics of learning, online learning and competitive analysis, hardness of learning and approximations, statistical theory, large deviations and asymptotic analysis, information theory. * Neuroscience: theoretical and experimental studies of processing and transmission of information in biological neurons and networks, including spike train generation, synaptic modulation, plasticity and adaptation. * Speech and Signal Processing: recognition, coding, synthesis, denoising, segmentation, source separation, auditory perception, psychoacoustics, dynamical systems, recurrent networks, language models, dynamic and temporal models. * Visual Processing: biological and machine vision, image processing and coding, segmentation, object detection and recognition, motion detection and tracking, visual psychophysics, visual scene analysis and interpretation. Demonstrations and Workshops: There is a separate Demonstration track at NIPS. Authors wishing to submit to the Demonstration track should consult the Call for Demonstrations. The workshops will be held at Lake Tahoe, Nevada, December 9-10. The upcoming call for workshop proposals will provide details. Web URL: https://nips.cc/Conferences/2013/CallForPapers
SIAM international conference on Data Mining (SDM) - 17 septembre 2012 - PUBLICATION
http://www.siam.org/meetings/sdm13/
October 12, 2012 11:59 PM PST: | Paper Submission* |
December 20, 2012: | Author Notification |
2013 ACM SIGMOD/PODS @ New York, New York, USA - 29 août 2012 - PUBLICATION
Important Dates
SIGMOD Deadlines
November 13, 2012: Submission deadline for papers February 5, 2013: Notification of acceptance, rejection, revision March 5, 2013: Submission deadline for revised papers April 9, 2013: Notification of acceptance, rejection for revised papers April 16, 2013: Camera-ready deadline
PODS Deadlines
Dec 5, 2012: Submission deadline for papers Feb 25, 2013: Notification of acceptance, rejection
The 17th Pacific-Asia Conference on Knowledge Discovery and Data Mining - 12 août 2012 - PUBLICATION
FREE ACM Learning Webinar, June 28: "2012 - Big Data: End of the World or End of BI?" - 6 juin 2012 - PUBLICATION
Registration link
expose - 31 mai 2012 - PUBLICATION
expose - 31 mai 2012 - PUBLICATION
Extraction de quadri-concepts à partir de d-folksonomies : Application à la détection de tendances - 31 mai 2012 - PUBLICATION
Cet exposé présente une étude théorique sur l'approche quadratique effectuée sur les folksonomies pour l'extraction de quadri-concepts. Ces structures sont des quadruplets d'utilisateurs, tags, ressources et dates. Un algorithme appelé QuadriCons a été proposé pour permettre une telle extraction. Dans une deuxième partie, nous analysons les folksonomies à travers une détection de tendances sur les bases MovieLens et Last.FM afin de mettre en avant l'utilité des quadri-concepts.
Évolution de la stabilité de la sélection de variables en fonction de la taille d’échantillon et de la dimension - 30 mai 2012 - PUBLICATION
This paper appeared in CAP 2012 Résumé : La sélection de variables est une étape importante lors de la construction d’un classificateur sur des données de grande dimension. Lorsque le nombre d’observations est faible, cette sélection a tendance à être instable, au point qu’il est courant d’observer que sur deux jeux de données différents mais traitant d’un problème similaire, les variables sélectionnées ne se recoupent presque pas. Pourtant, ce problème de la stabilité semble encore peu étudié. Dans cet article, nous présentons des méthodes de quantification de la stabilité, puis nous en étudions les variations en fonction de divers paramètres (dimensionalité, nombre d’observations, distribution des variables, seuil de sélection) sur des données artificielles, avant de réaliser ces mesures sur des données réelles d’expression génique (données puces). Mots-clés : Sélection de variables, stabilité, petits échantillons.
How to Write and Publish in Knowledge & Data Engineering - 20 février 2012 - PUBLICATION
How to Write and Publish in Knowledge & Data Engineering
Writing09-Web.pdf
How to Write and Publish in Knowledge & Data Engineering - 20 février 2012 - PUBLICATION
How to Write and Publish in Knowledge & Data Engineering
Events - 2 février 2012 - PUBLICATION
This page is used to view your full list of events and event details.
Manage this page as any other, except:
- DO NOT delete this page while IZ-Calender is in use.
- DO NOT change the slug of this page from here, use Admin menu-> IZ-Calender -> Settings -> Set the events page slug
Go to: http://codex.wordpress.org/Pages#Changing_the_URL_.28or_.22Slug.22.29_of_Your_Pages to read more on page slugs. - DO NOT edit this page's status or Visibility
- To remove this page from your navigation structure go to Admin menu-> IZ-Calender -> Settings -> Display this page in my primary nav -> Select "No"
- The information you see here will not display in your theme (user-interface)
Frequent subgraph selection by means of substitution matrix - 31 janvier 2012 - PUBLICATION
Frequent subgraph selection by means of substitution matrix
DDSMG.pdf
Thursday. 09 September 2021, 2:00 pm
Talk 1: Andressa's internship pre-defense
Talk 2: Abdoul's Ph.D. pre-defense
NeuroDeRisk project online meeting, October 13-14, 2021
Award: EDA challenge 2021
First meeting after the 2021 summer break
Miner Team
Poster from the DAPPEM project
Miners team during the Covid19